Popular search categories
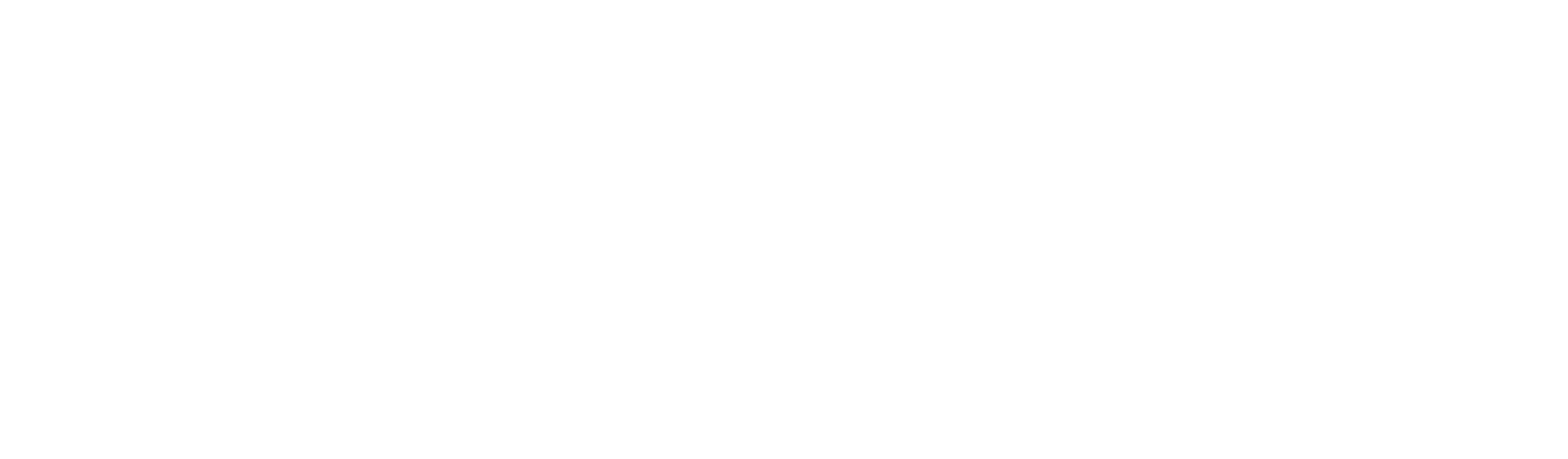
Company overview
Lenz Labs develops artificial intelligence (AI) based solutions to manage rail asset risks and improve performance and reliability of the UK’s rail networks.
Our software leverages machine learning and infrastructure data to support rail operators with maintenance and scheduling decisions to increase the efficiency, safety, and capacity of passenger and freight services.
Solutions
We focus on maximizing the performance of technical assets and operational teams at the wheel-rail interface.
Valorizing network visibility
Network operations and maintenance involve decisions based on large numerical and language-based data sets.
From naïve models to deep reinforcement learning, Lenz Labs solutions amplify weak signals from diverse data sources into actionable insights. We then contextualize predictions to help rail achieve the reliability of a true mobility solution. Starting with track condition monitoring for managing weather-related risks (seasonal delivery), Lenz Labs is empowering operations and scheduling teams to turn weak signals from disparate data sources into actionable insights to meet ambitious safety and performance targets.
Achieving distributed reliability
Low rolling resistance gives rail the comparative energy efficiency advantage over road transport. But unreliable track conditions can mean speed restrictions are imposed to guarantee braking within safe distances on the network. Speed changes on one service will often affect other passenger and freight services across the route and regions.
Existing mitigation measures are only partially efficient. This is why we have designed a train-based solution to diagnose and mitigate poor track condition without the wait for maintenance crews.
Exploring new data frontiers
Our team delivers next generation sensors. Our development and testing facilities are based at the heart of the Edinburgh start-up ecosystem at Codebase Edinburgh. As part of our R&D activities, the team has implemented a wheel slide protection system on our own train, iterating on existing designs to deliver a system capable of detecting a range of challenges at the wheel-rail interface, far beyond the existing sensing capability on the market.
Seasonal Delivery Portal
Weather-induced low-adhesion conditions reduce a train’s ability to brake and accelerate efficiently. It’s costly and in extreme cases with multiple concurrent failures, such as in the Salisbury 2019 incident, unmitigated low adhesion can even lead to train derailments.
The Lenz Labs Portal combines historical and live data sources with a series of Machine Learning models to empower seasonal delivery specialists to plan better mitigation strategies ahead of low adhesion affecting network service. Advanced visualisation, prediction, and recommendations contribute to maturing seasonal capability from a condition-based to a predictive level capability.
Delivery driven by forecasted risk
We use Machine Learning to enable operators to transition from reactive to predictive operations.
- Monitor asset degradation – plan for maintenance before failure occurs.
- Identify risk – view live data to stay on top of emerging delay risks.
- Prioritise mitigation – choose the optimal scenario amongst alternatives and monitor its execution.
- Benchmark performance – evaluate the effectiveness of your interventions.
Traction Hub
Low adhesion remains a source of unfortunate safety incidents, with existing planning and mitigation measures only partially efficient. We have designed a train-based solution to sense and de-risk the track condition before it is an issue.
Think of the Traction Hub as an ABS for trains. It generates an instant recovery of normal braking conditions for any vehicle equipped with it, independent of the actual track condition. Protecting the service and the network from schedule impact. We have performed a full-scale static test on our own Class 19 ex-DVT vehicle. Having shown a 20% increase in downforce, we are now looking to partner with ROSCOs and network owners for further low and high-speed testing and to develop the strategic case for a network operator mandate.